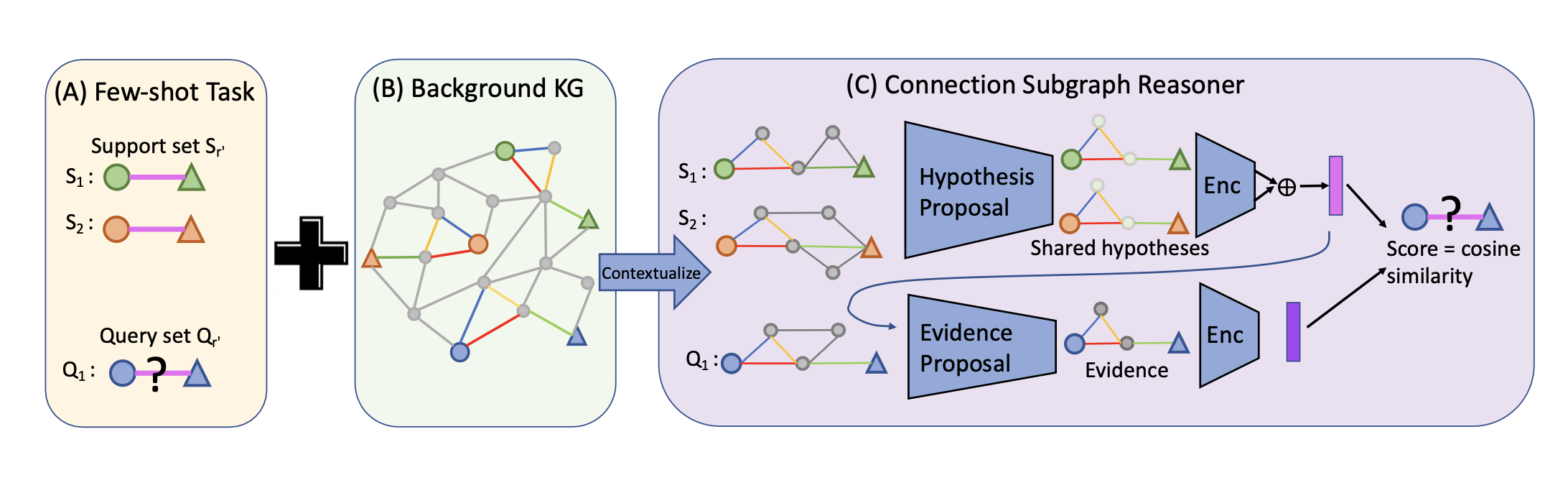
はじめに
- NeurIPS22
- Few-shotにおける knowledge graph completion task を行う
- 上図のように, Background KG (knowledge graph)とsupport setが与えられた状態で, Query setのrelationを推論するタスク
- Connection Subgraph Reasoner (CSR)を提案
- Few-shotにおける knowledge graph completion task を行う
Few-shot KG Completion
-
KGは
で表される- ここで,
はそれぞれentityとrelationで, はtripletで
- ここで,
-
Few-shotknowledge graph completion taskとは
なような について (=unseenなrelationについて)- support set
が与えられた状態で の"?“に該当するentityを当てるタスク
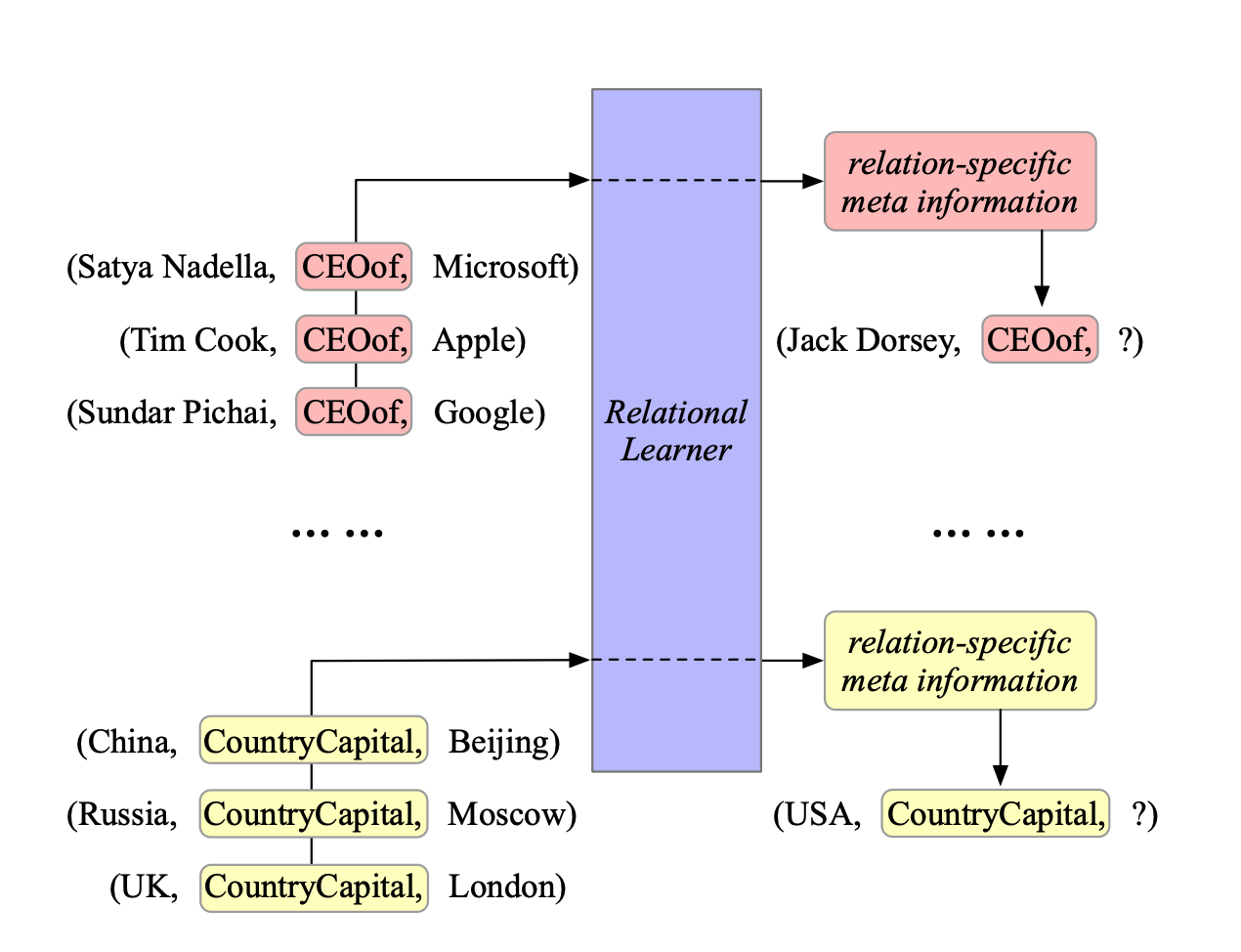
引用: M. Chen, W. Zhang, W. Zhang, Q. Chen, and H. Chen. Meta relational learning for few-shot link prediction in knowledge graphs. In EMNLP, 2019.
Inductive Reasoning
-
以下のようなものをhypothesisと呼ぶ
-
基本的には有効なhypothesisを探すことを目的とする
General Framework
- まずは一般化されたフレームワークを提案している
- このフレームワークに則り,Learning-freeな方法(CSR-OPT)と学習可能な方法(CSR-GNN)の二つを提案している
- Triplet Contextualization
- support setとクエリのTripletに対して, background KGからサブグラフを作成する
- 具体的には, ノードからk-hopで到達しうるノードまでを使ってサブグラフを構成する
- これにより知識グラフによって"contextualized"されたグラフを得ることができる
- Hypothesis Proposal
- 一般にhypothesis proposal module と呼ばれる
を用いて, support setに対する全てのサブグラフに対してhypothesisを提案する はどのエッジを採用するかを示すsoft edge mask を出力する- エッジを採用 = tripletを採用
- ただし,
は以下の式を満たす必要がある ( はcosine類似度)
- Hypothesis Testing.
-
一般にevidence proposal module
と呼ばれる を用いてhypothesisが正しいかどうかを検証する
-
ただし
は以下の式を満たす必要がある ( はcosine類似度)
CSR-OPT (Learning-free)
-
hypothesis proposal module
について, 以下の最適化問題を解く
- ただし,
は を隣接行列, として, 以下の通り
- おそらく
なので とか出てくる
- ただし,
Aが無向または有向グラフGの隣接行列とすると、行列An(すなわちAのn個の複製の積)は興味深い解釈を持つ: 要素(i, j)は頂点iから頂点jへの長さnの(有向または無向)歩道の数を与える。nが、あるi、jについてAnの要素(i, j)が正となるような最小の非負整数とすると、nは頂点iと頂点jとの間の距離である。
引用: Wikipedia ─ 隣接行列
-
evidence proposal module
について, 以下の最適化問題を解く ( は正則化項)
はランダムなGNN (要検証)
CSR-GNN
, として, それぞれを , とする にはPathConを使用- Relational Message Passing for Knowledge Graph Completion (KDD21)
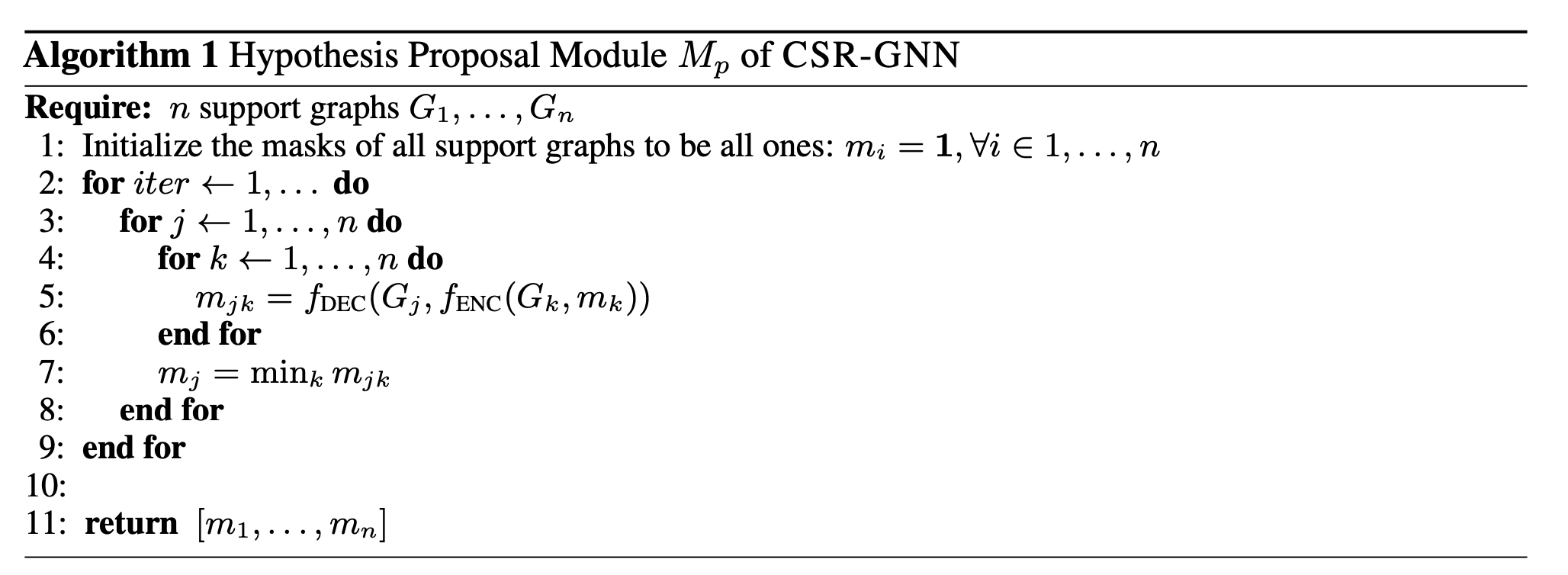
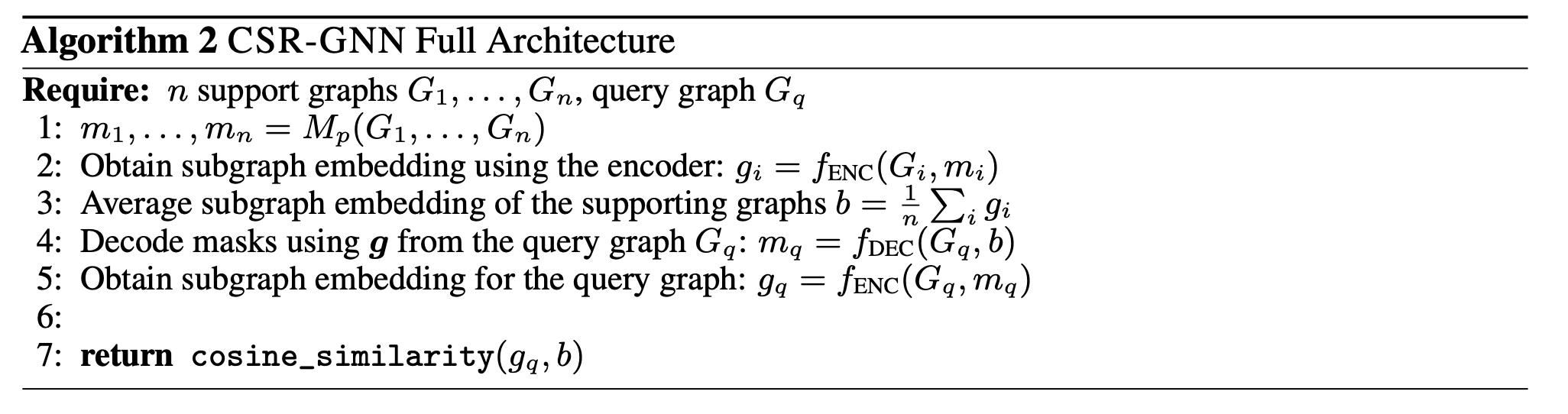
- 学習方法
-
reconstruction loss とcontrastive lossを使用
-
に関して, -
同じrelationに対して異なるtripetをサンプリングしてきたものを
として正例と負例を作る
-
実際の推論例
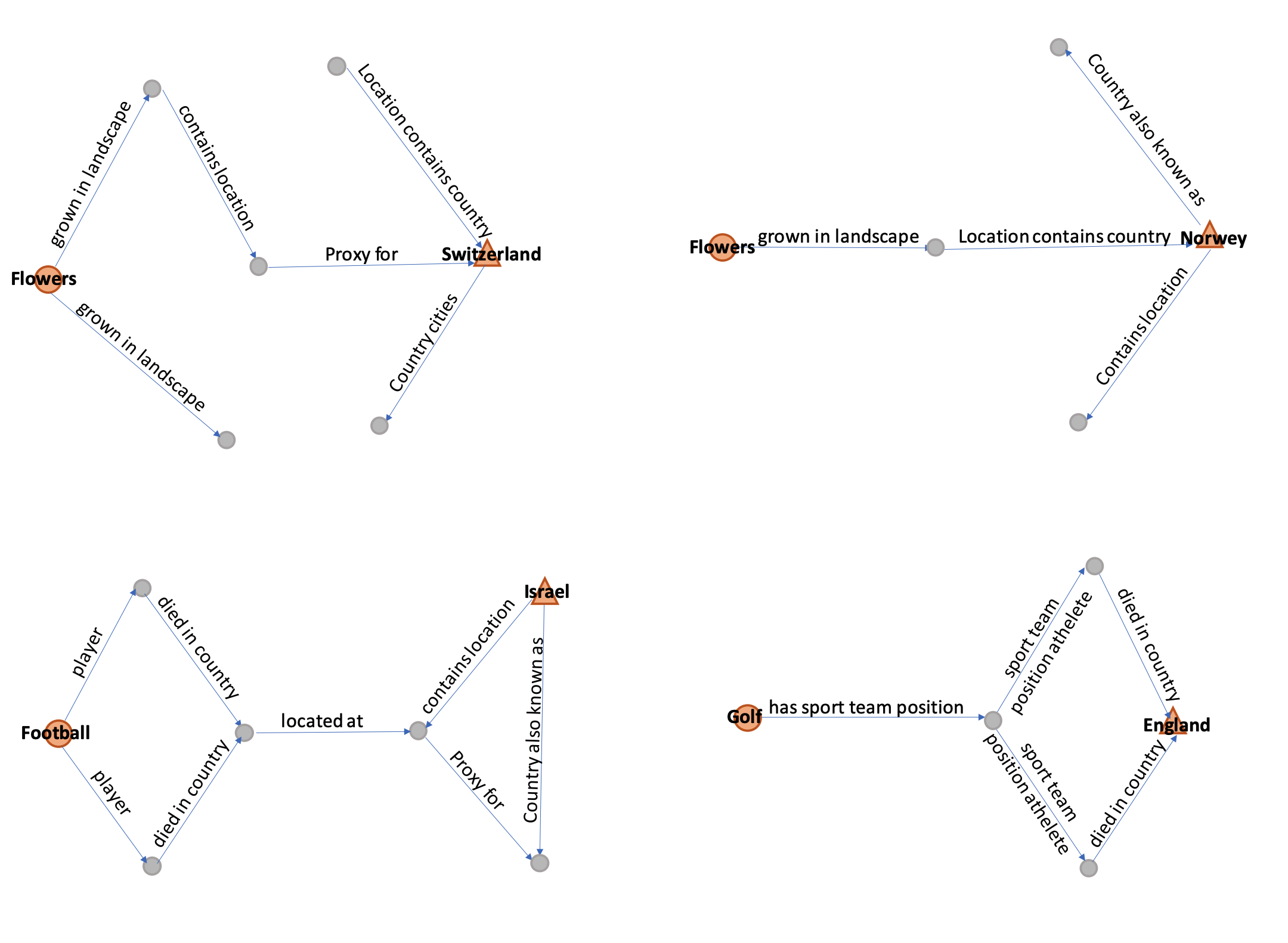